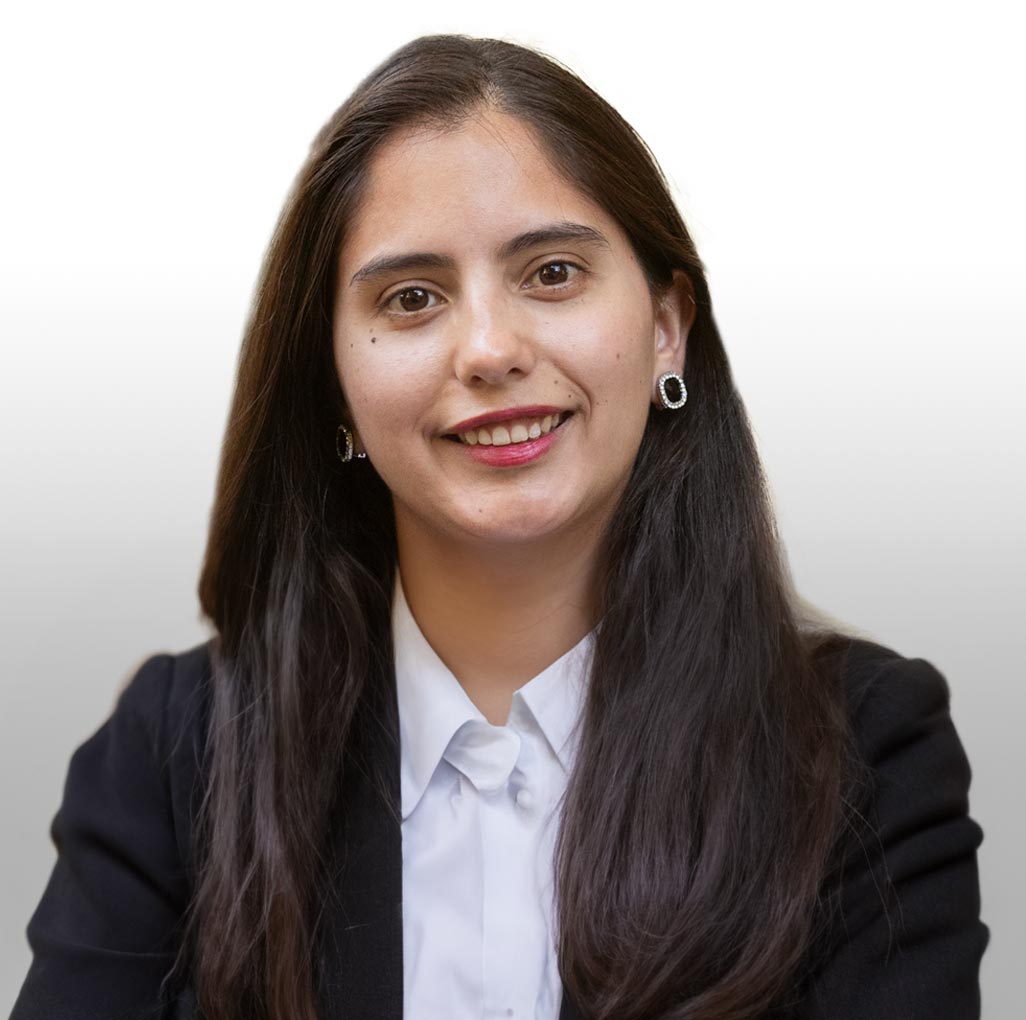
By: Paola Ruiz
Data Scientist
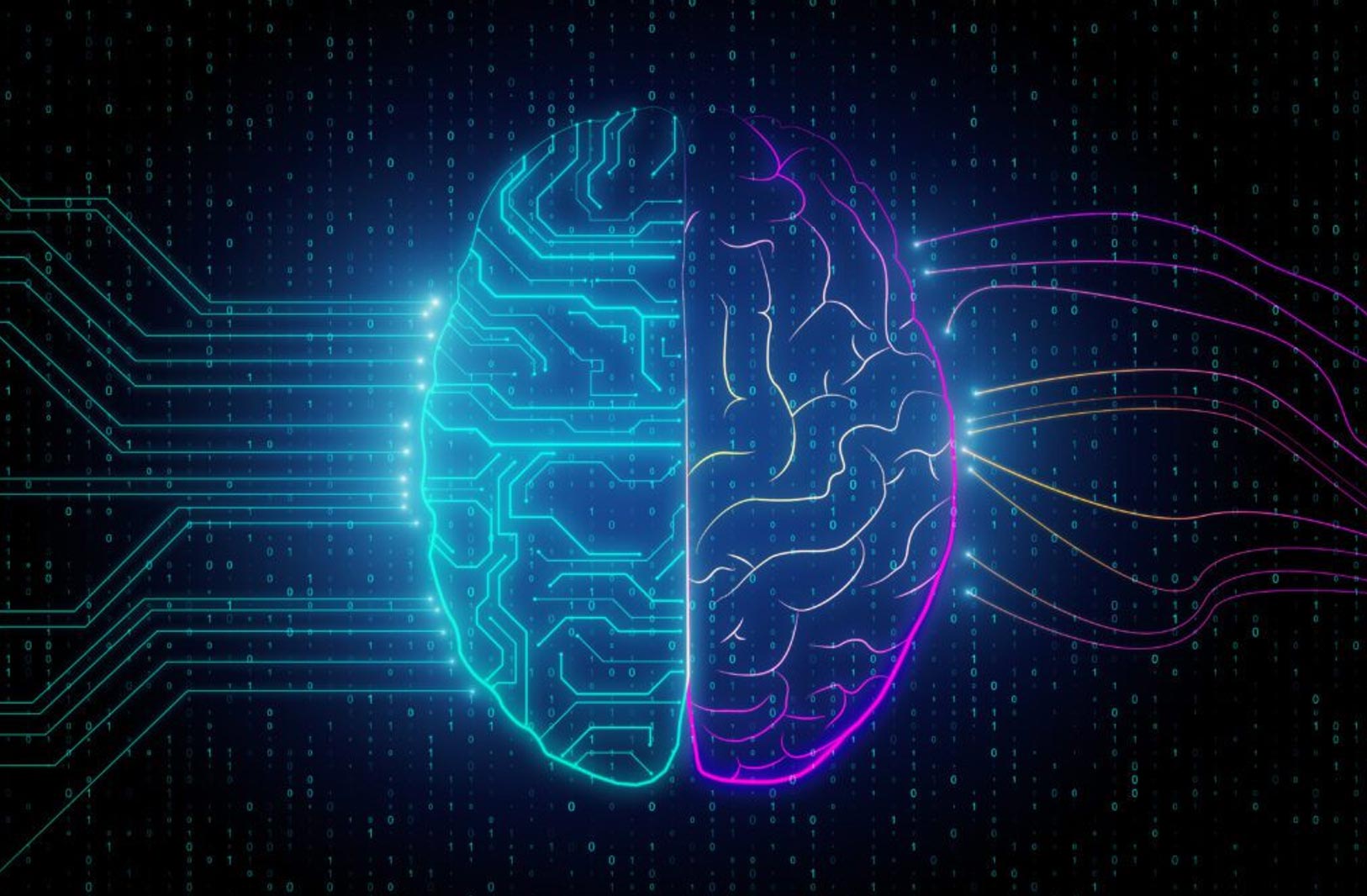
Regarding diagnosis, AI’s capacity to analyze vast and complex datasets enables the identification of previously unknown risk factors. By integrating diverse data types, including genetic, sociodemographic, health information, lifestyle, and neuroimaging data, AI algorithms can discern intricate patterns and correlations, contributing to a more comprehensive understanding of AD risk profiles while combining biological and non-biological markers.13 For instance, longitudinal studies like ELSA, MHAS, CHARLS, LASI, HAALSI, and HRS that have in-depth cognitive studies enable the assessment of the interplay between social and environmental factors, lifestyle choices, and other health conditions for the development of AD by comparing subjects with similar backgrounds but different diagnosis. This analysis might enable distinguishing pathological aging from normal aging with unprecedented precision.
Furthermore, considering that brain changes start occurring years before symptoms arise,14 AI can also enhance early detection of AD by analyzing subtle variations that might be imperceptible to the human eye. In this sense, datasets such as NACC, DIAN, ADNI, EPAD, and AMYPAD, among others, that contain multimodal data like various types of images, biomarkers, cognition scales, genetic and environmental descriptions, are highly suitable to develop the AI models.15,16 Moreover, AI’s predictive power can extend to forecasting the disease progression of patients.17-,19 In the future, one of the most anticipated outcomes of AI in health is to help deliver precision medicine, and the tasks mentioned above are steps towards that goal.20,21
Within clinical trials, AI can refine site selection for sponsors and enhance participant matching and enrollment by ensuring a more accurate fit between trial requirements and participant profiles.22 Additionally, it can help in protocol design, utilizing predictive models to foresee potential challenges and early terminations, enabling protocol modification to improve its probability of success.23 Moreover, AI’s impact extends to data analysis, going beyond traditional statistical methods. Since it can easily handle complex, multimodal, multi-dimensional data sets, AI algorithms can uncover insights that might not be evident through standard analysis techniques. This ability ensures a deeper understanding of trial results and enables the discovery of novel pathways and new therapeutic interventions.
At IGC Pharma, we are using AI to improve our clinical trial data analysis. We seek to gain a deeper understanding of the factors influencing differential treatment responses among participants. Identifying those factors will aid in pinpointing the specific patient population that should be targeted. Through AI, we can uncover patterns and biomarkers faster, leading to earlier diagnosis and tailored therapies. This technology also accelerates drug development, promising better understanding and treatment of AD for improved patient outcomes.
- Marr, B. (2019, December 16). The 10 best examples of how AI is already used in our everyday life. Forbes, https://www.forbes.com/sites/bernardmarr/2019/12/16/the-10-best-examples-of-how-ai-is-already-used-in-our-everyday-life/
- McKinsey Explainers. (2023, January 19). What is Generative AI?. McKinsey & Company. https://www.mckinsey.com/featured-insights/mckinsey-explainers/what-is-generative-ai
- Siemens Healthineers. (2023, September 12). How AI is transforming the field of radiology. Harvard Business Review. https://hbr.org/sponsored/2023/09/how-ai-is-transforming-the-field-of-radiology
- (2022, November 24). 10 real-world examples of AI in Healthcare. Philips, https://www.philips.com/a-w/about/news/archive/features/2022/20221124-10-real-world-examples-of-ai-in-healthcare.html
- Office of the Commissioner. (2021, September 21). FDA authorizes software that can help identify prostate cancer. U.S. Food and Drug Administration. https://www.fda.gov/news-events/press-announcements/fda-authorizes-software-can-help-identify-prostate-cancer
- Kumar, Y., Koul, A., Singla, R., & Ijaz, M.F. (2022). Artificial Intelligence in disease diagnosis: A systematic literature review, synthesizing framework and future research agenda.Journal of Ambient Intelligence and Humanized Computing, 14(7), pp. 8459–8486. doi:10.1007/s12652-021-03612-z
- Chun, M. (2023, March 20). How artificial intelligence is revolutionizing drug discovery. Bill of Health – The blog of the Petrie-Flom Center at Harvard Law School, https://blog.petrieflom.law.harvard.edu/2023/03/20/how-artificial-intelligence-is-revolutionizing-drug-discovery/
- Kupietzky, J. (2023, September 28). The AI Revolution Reaches Healthcare Administration. Newsweek, https://www.newsweek.com/ai-revolution-reaches-healthcare-administration-1827401
- (n.d.). AI in Remote Patient Monitoring: The top 4 use cases in 2023. HealthSnap Inc. https://healthsnap.io/ai-in-remote-patient-monitoring-the-top-4-use-cases-in-2023/
- Fang, J., Zhang, P., Wang, Q., Chiang, C. W., Zhou, Y., Hou, Y., Xu, J., Chen, R., Zhang, B., Lewis, S. J., Leverenz, J. B., Pieper, A. A., Li, B., Li, L., Cummings, J., & Cheng, F. (2022). Artificial intelligence framework identifies candidate targets for drug repurposing in Alzheimer’s disease. Alzheimer’s research & therapy, 14(1), 7. https://doi.org/10.1186/s13195-021-00951-z
- Rodriguez, S., Hug, C., Todorov, P., Moret, N., Boswell, S. A., Evans, K., Zhou, G., Johnson, N. T., Hyman, B. T., Sorger, P. K., Albers, M. W., & Sokolov, A. (2021). Machine learning identifies candidates for drug repurposing in Alzheimer’s disease. Nature communications, 12(1), 1033. https://doi.org/10.1038/s41467-021-21330-0
- Jia, L., & Gao, H. (2022). Machine Learning for In Silico ADMET Prediction. Methods in molecular biology (Clifton, N.J.), 2390, 447–460. https://doi.org/10.1007/978-1-0716-1787-8_20
- Tan, W. Y., Hargreaves, C., Chen, C., & Hilal, S. (2023). A Machine Learning Approach for Early Diagnosis of Cognitive Impairment Using Population-Based Data. Journal of Alzheimer’s disease : JAD, 91(1), 449–461. https://doi.org/10.3233/JAD-220776
- National Institute on Aging. (2023, April 15). Alzheimer’s disease fact sheet. https://www.nia.nih.gov/health/alzheimers-and-dementia/alzheimers-disease-fact-sheet
- Venugopalan, J., Tong, L., Hassanzadeh, H. R., & Wang, M. D. (2021). Multimodal deep learning models for early detection of Alzheimer’s disease stage. Scientific reports, 11(1), 3254. https://doi.org/10.1038/s41598-020-74399-w
- El-Sappagh, S., Alonso, J. M., Islam, S. M. R., Sultan, A. M., & Kwak, K. S. (2021). A multilayer multimodal detection and prediction model based on explainable artificial intelligence for Alzheimer’s disease. Scientific reports, 11(1), 2660. https://doi.org/10.1038/s41598-021-82098-3
- Wang, C., Li, Y., Tsuboshita, Y., Sakurai, T., Goto, T., Yamaguchi, H., Yamashita, Y., Sekiguchi, A., Tachimori, H., & Alzheimer’s Disease Neuroimaging Initiative (2022). A high-generalizability machine learning framework for predicting the progression of Alzheimer’s disease using limited data. NPJ digital medicine, 5(1), 43. https://doi.org/10.1038/s41746-022-00577-x
- Beheshti, I., Geddert, N., Perron, J., Gupta, V., Albensi, B. C., Ko, J. H., & Alzheimer’s Disease Neuroimaging Initiative (2022). Monitoring Alzheimer’s Disease Progression in Mild Cognitive Impairment Stage Using Machine Learning-Based FDG-PET Classification Methods. Journal of Alzheimer’s disease : JAD, 89(4), 1493–1502. https://doi.org/10.3233/JAD-220585
- Al Olaimat, M., Martinez, J., Saeed, F., Bozdag, S., & Alzheimer’s Disease Neuroimaging Initiative (2023). PPAD: A deep learning architecture to predict progression of Alzheimer’s disease. bioRxiv : the preprint server for biology, 2023.01.28.526045. https://doi.org/10.1101/2023.01.28.526045
- CHIBA UNIVERSITY. (n.d.). Advancing precision medicine using AI and big data. Nature news. https://www.nature.com/articles/d42473-020-00349-9
- Ray, A. (2023, October 13). Council post: Ai renaissance in healthcare: Top five opportunities in precision medicine. https://www.forbes.com/sites/forbestechcouncil/2023/10/13/ai-renaissance-in-healthcare-top-five-opportunities-in-precision-medicine/?sh=dde55186a617
- Oikonomou, E. K., Thangaraj, P. M., Bhatt, D. L., Ross, J. S., Young, L. H., Krumholz, H. M., Suchard, M. A., & Khera, R. (2023). An explainable machine learning-based phenomapping strategy for adaptive predictive enrichment in randomized clinical trials. NPJ digital medicine, 6(1), 217. https://doi.org/10.1038/s41746-023-00963-z
- Kavalci, E., & Hartshorn, A. (2023). Improving clinical trial design using interpretable machine learning based prediction of early trial termination. Scientific reports, 13(1), 121. https://doi.org/10.1038/s41598-023-27416-7